Why is Data Insight So Important?
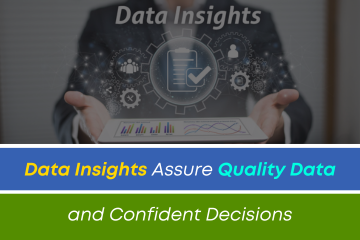
Every business (large or small) creates and depends upon data. One hundred years ago, businesses looked to leaders and experts to strategize and to create operational goals. Decisions were based on opinion, guesswork and a complicated mixture of notes and records reflecting historical results that might or might not be relevant to the future.
Today, organizations look to data and to technology to help them understand historical results, and predict the future needs of the enterprise to manage everything from suppliers and supplies to new locations, new products and services, hiring, training and investments. But too much data can also create issues. If the data is not easily gathered, managed and analyzed, it can overwhelm and complicate decision-makers.
‘Data insight techniques provide a comprehensive set of tools, data analysis and quality assurance features to allow users to identify errors, enhance data quality, and boost productivity.’
By some estimates, bad data costs global organizations more than five trillion USD annually.
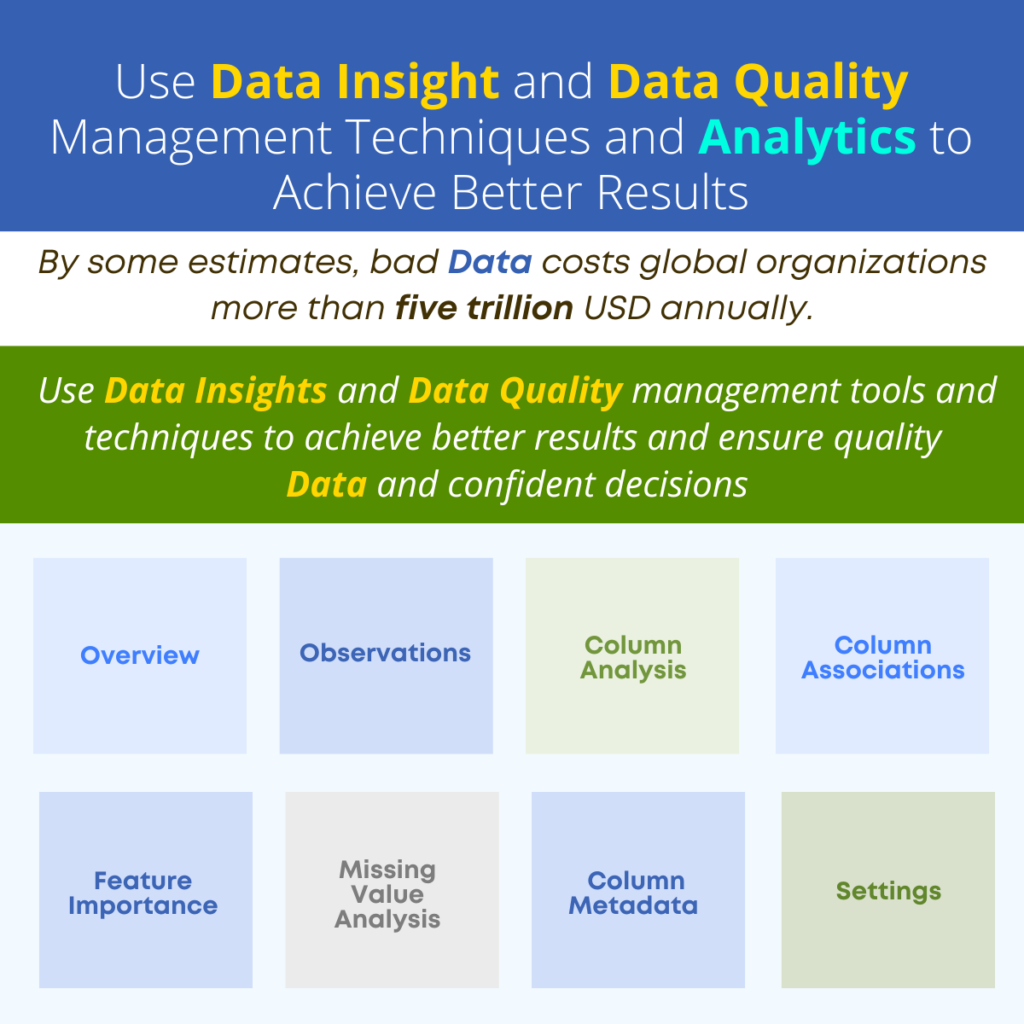
Data insight takes data to the next level by providing comprehensive data analysis and quality assurance features that empower business analysts and users to quickly and easily identify errors, enhance data quality, and boost productivity. The business can harness the power of statistics and machine learning to uncover those crucial nuggets of information that drive effective decision, and to improve the overall quality of data.
By incorporating machine learning, natural language processing and automation within advanced analytics solutions, the enterprise can improve results and support its team with augmented analytics that are designed as self-serve solutions for business users, so the team can gather and analyze information with ensured, sustained data quality and results that are clear and concise. When an analytics solution is built upon this foundation, with advanced tools and techniques to support users, the enterprise can ensure user adoption and positive outcomes. Users do not have to learn complex systems or look to data scientists or business analysts for answers.
Tools that support data insight include numerous data quality management techniques. These tools allow users to see and work with datasets in a way that is targeted and provides clear, actionable information for decisions and strategies.
Overview – Reveals the data quality index in percentage representing the quality level of data. It shows the quality of the dataset and number of columns with listing down the missing values, duplicates, and measure and dimension columns.
Observations – Highlights all detected inconsistencies and anomalies within your dataset, along with the corresponding column names. By clicking on a column name, you can access detailed information about the observation for that particular column and view recommendations for fixing the issue.
Column Analysis – Shows the details related to all the columns in the dataset. It categorizes the columns by their types and shows Sample values, Missing Values, Most frequent values, least frequent values, Unique values and Quality index of that column.
Column Associations – Shows the pairs associations between all columns which helps you to understand the relationship with each other. The degree of association can be determined by the index value, and higher the index indicating a stronger relationship between columns.
Feature Importance – Automatically identifies and displays the target variable along with its key predictors from your dataset. It also shows the influence of each predictor on the target. This helps you select the predictors that have the greatest impact, making it easier to create an effective predictive model.
Missing Value Analysis – Shows the analysis of the missing values across all the columns of the dataset at a glance. The graph visually represents both non-missing (non-null) values and missing (null) values, allowing you to quickly identify which columns have incomplete data.
Column Metadata – Provides information on the dataset’s recency, such as the last update and publication dates. It will also talk about the details like Datatype, Column Type and respective Sample Value of the columns in dataset.
Settings – Customize the data insights computing process for datasets to lower the load and processing time.
Data insight and Data Quality Management tools and techniques provide a comprehensive set of tools, data analysis and quality assurance features to allow users to identify errors, enhance data quality, and boost productivity. Users can uncover hidden insights and improve the overall quality of data with actionable recommendations to take prompt action.
‘By some estimates, bad data costs global organizations more than five trillion USD annually.’
To find out more about Natural Language Processing (NLP), Machine Learning And NLP Search Analytics, and comprehensive data quality management and Data Insight Tools, Contact Us. Discover the power of Augmented Analytics, machine learning, and Natural Language Processing (NLP). Read our free article, ‘Why Is Natural Language Processing Important To Enterprise Analytics?’.